Regularization for DNN
来源:互联网 发布:中央财经大学数据库 编辑:程序博客网 时间:2024/06/10 02:00
Regularization
There are several ways of controlling the capacity of Neural Networks to prevent overfitting:
L2 regularization is perhaps the most common form of regularization. It can be implemented by penalizing the squared magnitude of all parameters directly in the objective. That is, for every weight W += -lambda * W
towards zero.
L1 regularization is another relatively common form of regularization, where for each weight
Max norm constraints. Another form of regularization is to enforce an absolute upper bound on the magnitude of the weight vector for every neuron and use projected gradient descent to enforce the constraint. In practice, this corresponds to performing the parameter update as normal, and then enforcing the constraint by clamping the weight vector
Dropout is an extremely effective, simple and recently introduced regularization technique by Srivastava et al. in Dropout: A Simple Way to Prevent Neural Networks from Overfitting (pdf) that complements the other methods (L1, L2, maxnorm). While training, dropout is implemented by only keeping a neuron active with some probability
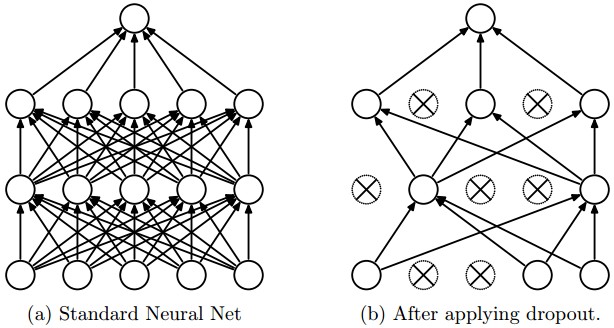
Vanilla dropout in an example 3-layer Neural Network would be implemented as follows:
""" Vanilla Dropout: Not recommended implementation (see notes below) """p = 0.5 # probability of keeping a unit active. higher = less dropoutdef train_step(X): """ X contains the data """ # forward pass for example 3-layer neural network H1 = np.maximum(0, np.dot(W1, X) + b1) U1 = np.random.rand(*H1.shape) < p # first dropout mask H1 *= U1 # drop! H2 = np.maximum(0, np.dot(W2, H1) + b2) U2 = np.random.rand(*H2.shape) < p # second dropout mask H2 *= U2 # drop! out = np.dot(W3, H2) + b3 # backward pass: compute gradients... (not shown) # perform parameter update... (not shown) def predict(X): # ensembled forward pass H1 = np.maximum(0, np.dot(W1, X) + b1) * p # NOTE: scale the activations H2 = np.maximum(0, np.dot(W2, H1) + b2) * p # NOTE: scale the activations out = np.dot(W3, H2) + b3
In the code above, inside the train_step
function we have performed dropout twice: on the first hidden layer and on the second hidden layer. It is also possible to perform dropout right on the input layer, in which case we would also create a binary mask for the input X
. The backward pass remains unchanged, but of course has to take into account the generated masks U1,U2
.
Crucially, note that in the predict
function we are not dropping anymore, but we are performing a scaling of both hidden layer outputs by
The undesirable property of the scheme presented above is that we must scale the activations by
""" Inverted Dropout: Recommended implementation example.We drop and scale at train time and don't do anything at test time."""p = 0.5 # probability of keeping a unit active. higher = less dropoutdef train_step(X): # forward pass for example 3-layer neural network H1 = np.maximum(0, np.dot(W1, X) + b1) U1 = (np.random.rand(*H1.shape) < p) / p # first dropout mask. Notice /p! H1 *= U1 # drop! H2 = np.maximum(0, np.dot(W2, H1) + b2) U2 = (np.random.rand(*H2.shape) < p) / p # second dropout mask. Notice /p! H2 *= U2 # drop! out = np.dot(W3, H2) + b3 # backward pass: compute gradients... (not shown) # perform parameter update... (not shown) def predict(X): # ensembled forward pass H1 = np.maximum(0, np.dot(W1, X) + b1) # no scaling necessary H2 = np.maximum(0, np.dot(W2, H1) + b2) out = np.dot(W3, H2) + b3
There has a been a large amount of research after the first introduction of dropout that tries to understand the source of its power in practice, and its relation to the other regularization techniques. Recommended further reading for an interested reader includes:
- Dropout paper by Srivastava et al. 2014.
- Dropout Training as Adaptive Regularization: “we show that the dropout regularizer is first-order equivalent to an L2 regularizer applied after scaling the features by an estimate of the inverse diagonal Fisher information matrix”.
Theme of noise in forward pass. Dropout falls into a more general category of methods that introduce stochastic behavior in the forward pass of the network. During testing, the noise is marginalized over analytically (as is the case with dropout when multiplying by
Bias regularization. As we already mentioned in the Linear Classification section, it is not common to regularize the bias parameters because they do not interact with the data through multiplicative interactions, and therefore do not have the interpretation of controlling the influence of a data dimension on the final objective. However, in practical applications (and with proper data preprocessing) regularizing the bias rarely leads to significantly worse performance. This is likely because there are very few bias terms compared to all the weights, so the classifier can “afford to” use the biases if it needs them to obtain a better data loss.
Per-layer regularization. It is not very common to regularize different layers to different amounts (except perhaps the output layer). Relatively few results regarding this idea have been published in the literature.
In practice: It is most common to use a single, global L2 regularization strength that is cross-validated. It is also common to combine this with dropout applied after all layers. The value of
- Regularization for DNN
- Regularization
- Regularization
- Regularization
- Regularization
- Proximal Gradient Descent for L1 Regularization
- Max-Margin Regularization for Chamfer Matching
- DNN
- Module development Template for DNN 7.0
- Sequence Training in DNN/CNN for ASR
- 【Stanford机器学习笔记】4-Regularization for Solving the Problem of Overfitting
- READING NOTE: Learning Spatial Regularization with Image-level Supervisions for Multi-label ...
- Learning Spatial Regularization with Image-level Supervisions for Multi-label Image Classification
- 5-Regularization
- Regularization Exercise
- ML_note:Regularization
- Task3 regularization
- Regularization (mathematics)
- C++常对象,常变量,长成员函数详解(转)
- 75. Sort Colors
- 567. Permutation in String
- HTML 5新增标签及CSS 3新增属性
- Java里重载的要求
- Regularization for DNN
- 80. Remove Duplicates from Sorted Array II
- 祭天时不同程序员的不同杀法
- 636. Exclusive Time of Functions
- 368. Largest Divisible Subset
- 208. Implement Trie (Prefix Tree)
- 机器学习课堂笔记6
- 代码生成代码,JavaBean Optional方式加强
- 单例模式解析